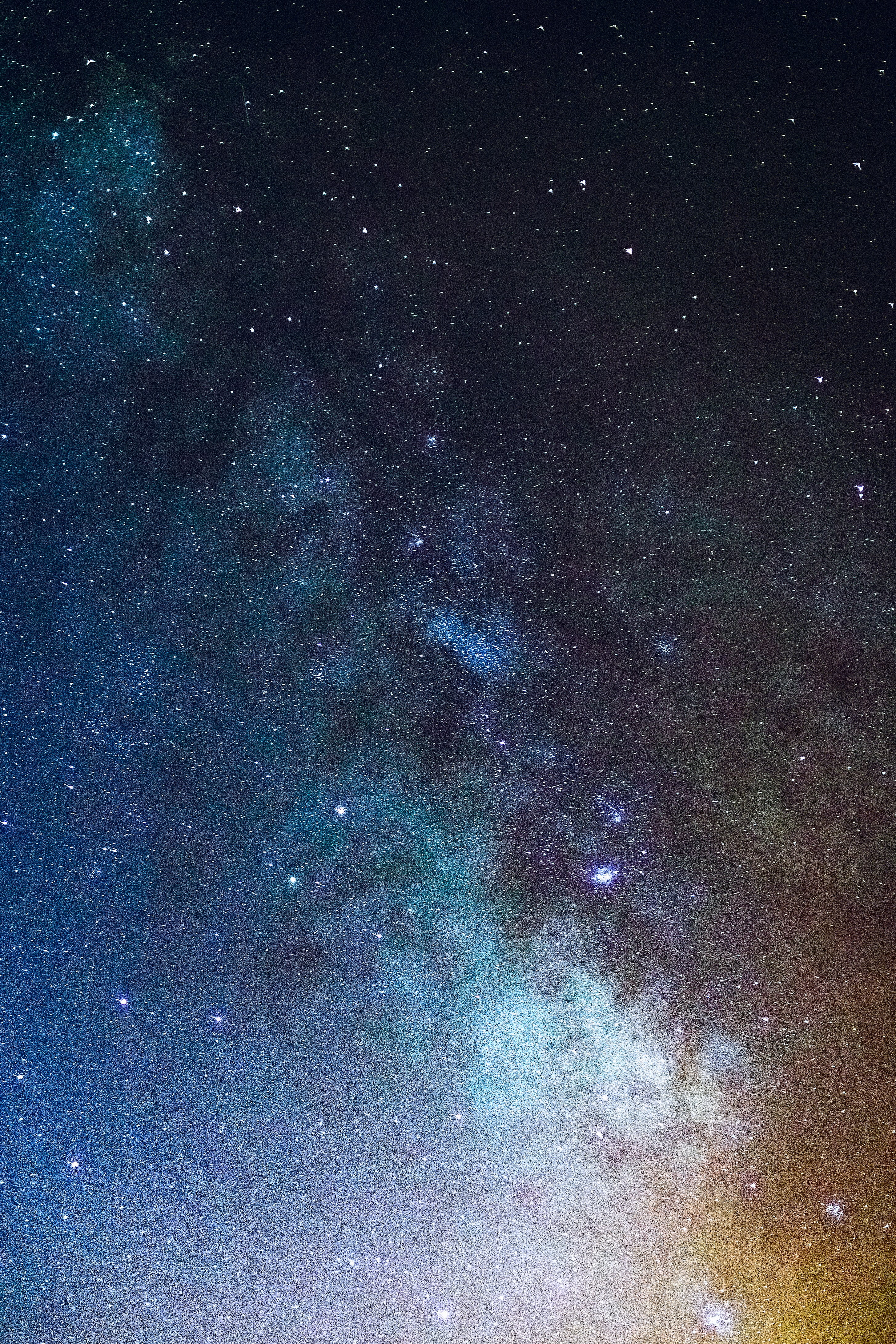
Overview
Artificial intelligence (AI) has the potential to become an engine for scientific discovery across disciplines – from predicting the impact of climate change, to using genetic data to create new healthcare treatments, and from finding new astronomical phenomena to identifying new materials here on Earth.
The Accelerate Programme for Scientific Discovery will advance the frontiers of science through the application of AI. Supported by a donation from Schmidt Futures, a philanthropic initiative founded by Eric and Wendy Schmidt, the Accelerate Programme will provide young researchers with specialised training in AI techniques, equipping them with the skills they need to use machine learning and AI to power their research.
By pursuing an ambitious research agenda that applies machine learning to the scientific challenges of the 21st century, the Programme will generate insights that accelerate scientific progress and create AI tools that are capable of delivering benefits for science and society.
Related People
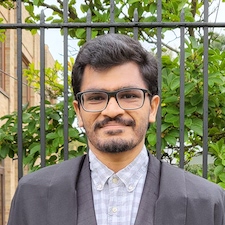
Aditya Ravuri
PhD Student, Cambridge University
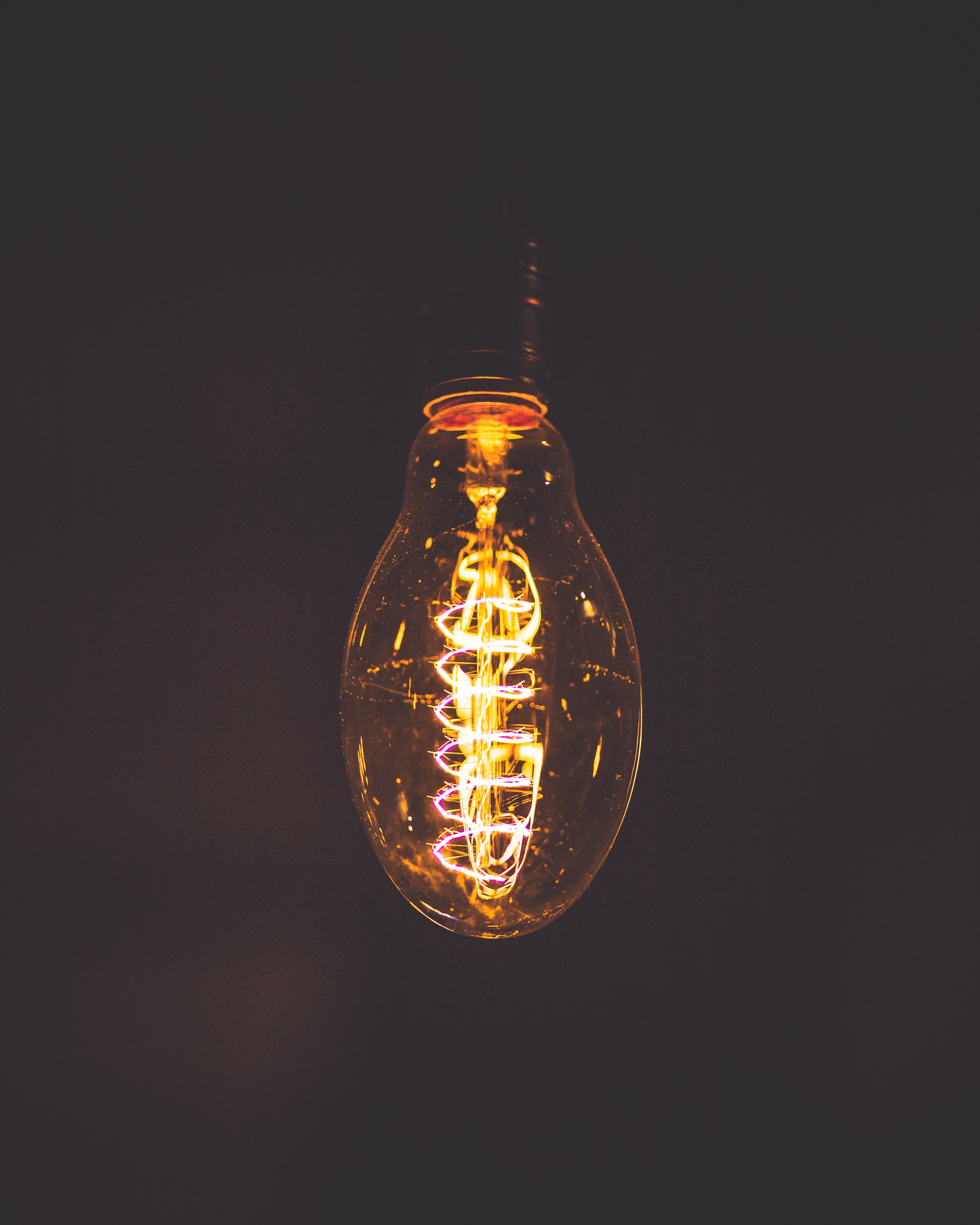
Ahmad Abu-Khazneh
Senior Machine Learning Engineer, Accelerate Programme, Cambridge University
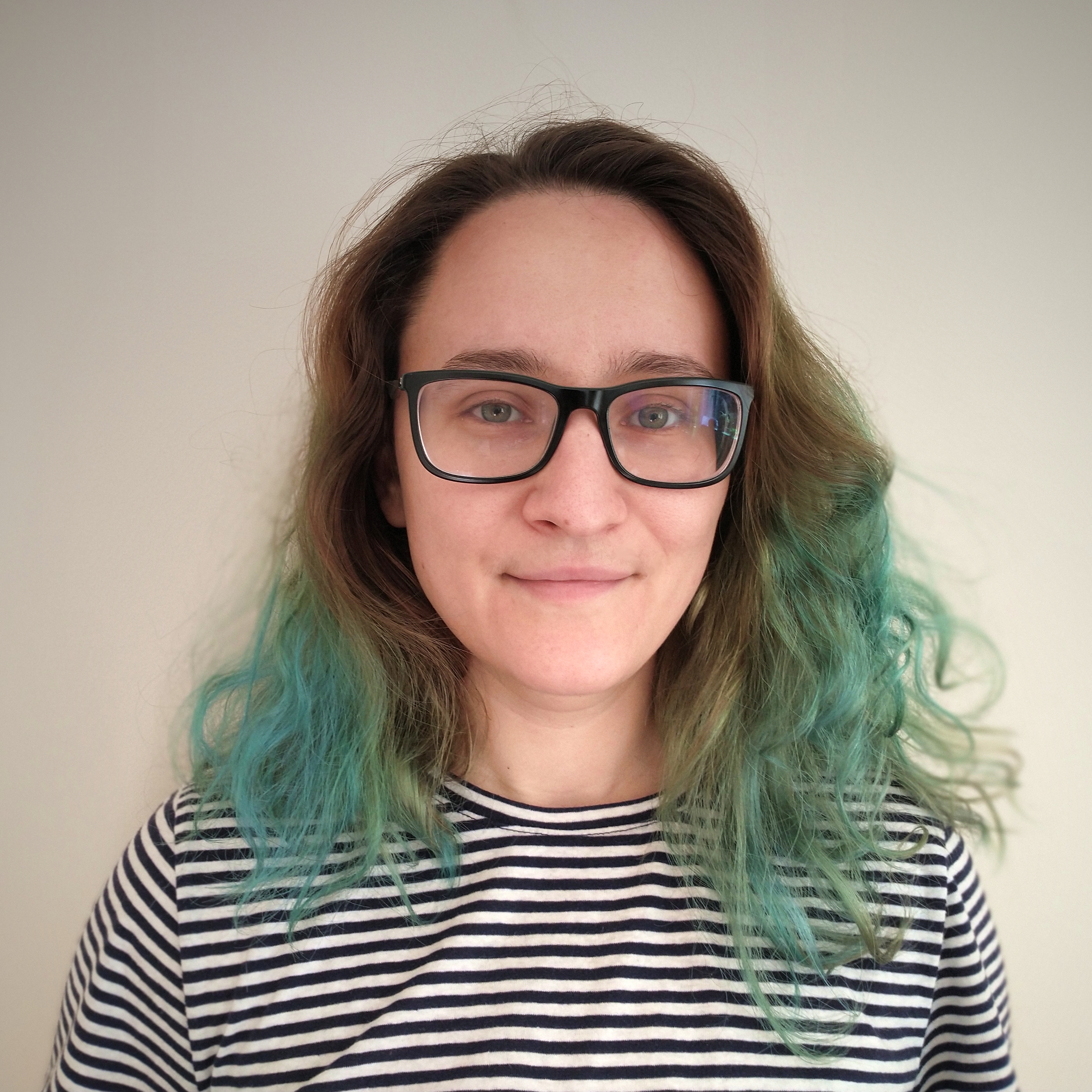
Bianca Dumitrascu
Departmental Early Career Academic Fellow, Accelerate Programme, Cambridge University
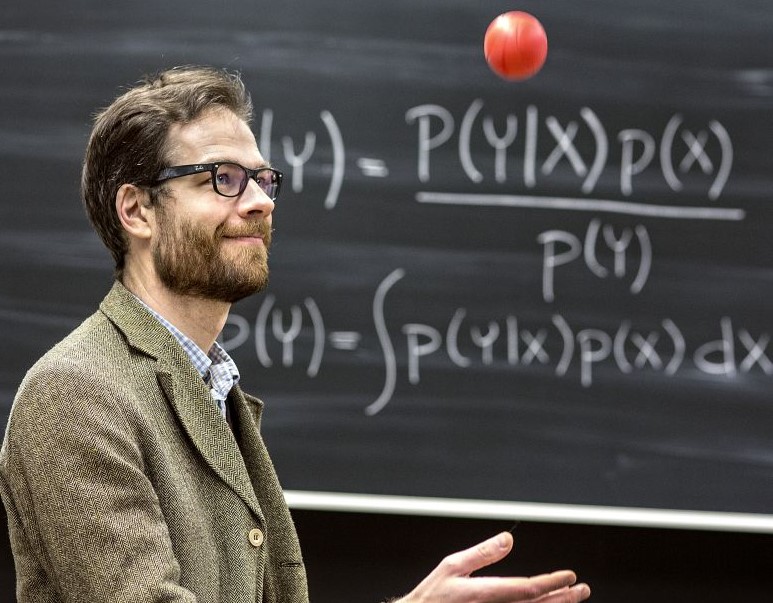
Carl Henrik Ek
Senior Lecturer, Cambridge University
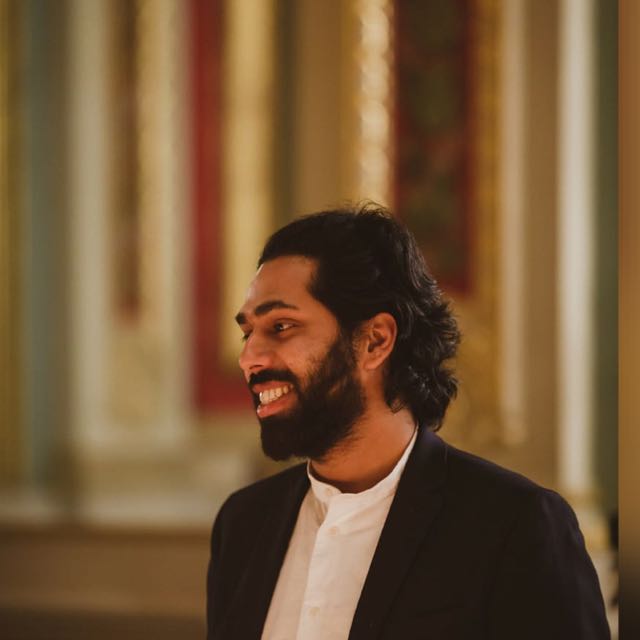
Challenger Mishra
Departmental Early Career Academic Fellow, Accelerate Programme, Cambridge University
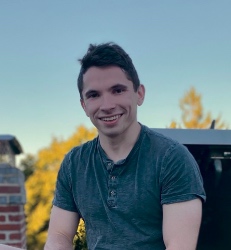
Isaac Sebenius
PhD Student, Cambridge University
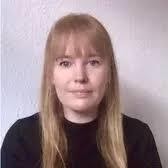
Jess Montgomery
Executive Director, Accelerate Science, Cambridge University
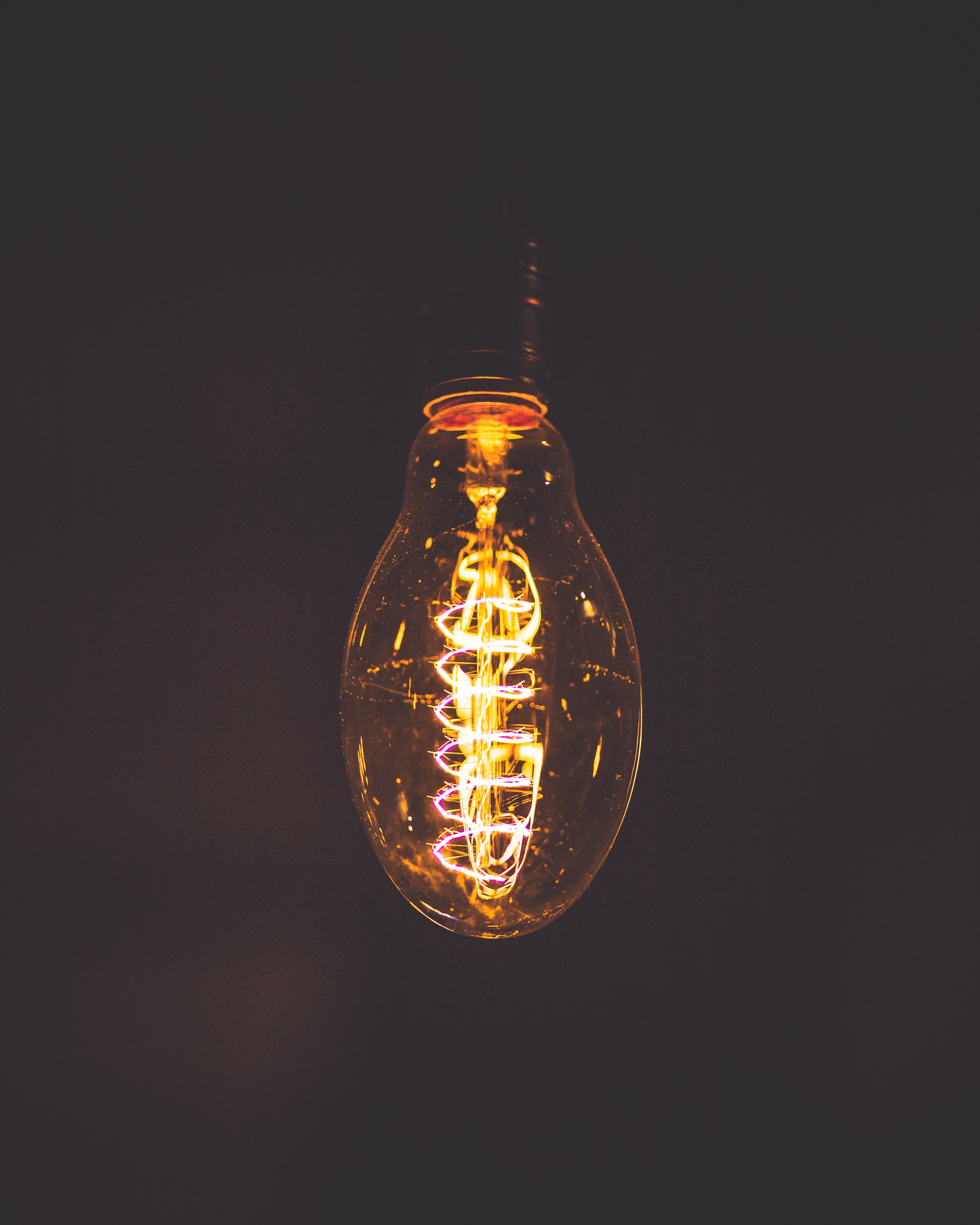
Justin Tan
PhD Student, Cambridge University
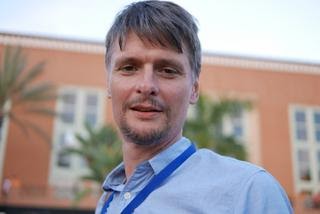
Neil D. Lawrence
The DeepMind Professor of Machine Learning, Cambridge University
Related Publications
Natural Language Processing markers in First Episode Psychosis and People at Clinical High-risk
Translational Psychiatry, 11(630):
Deep learning for Bioimage Analysis in Developmental Biology
Development, 148(18):
Optimal marker gene selection for cell type discrimination in single cell analyses
Nature Communications, 12(1186):
Solving Schrödinger Bridges via Maximum Likelihood
Entropy, 23(9):1134
Multimodal Graph Coarsening for Interpretable, MRI-Based Brain Graph Neural Network
IEEE 31st International Workshop on Machine Learning for Signal Processing (MLSP), :
Gaussian Process Latent Variable Flows for Massively Missing Data
Third Symposium on Advances in Approximate Bayesian Inference, :