AI deployment and systems design
A gap is emerging between our aspirations for the benefits of AI and our ability to deploy these technologies to tackle real-world challenges. Achieving the full potential of AI – and its benefits for society and the economy – requires the ability to safely and effectively deploy AI systems at scale. Research in this theme considers the advances in AI and system design that can manage the complex interactions that arise in real-world applications, from innovations in statistical emulation to software engineering for machine learning deployment.
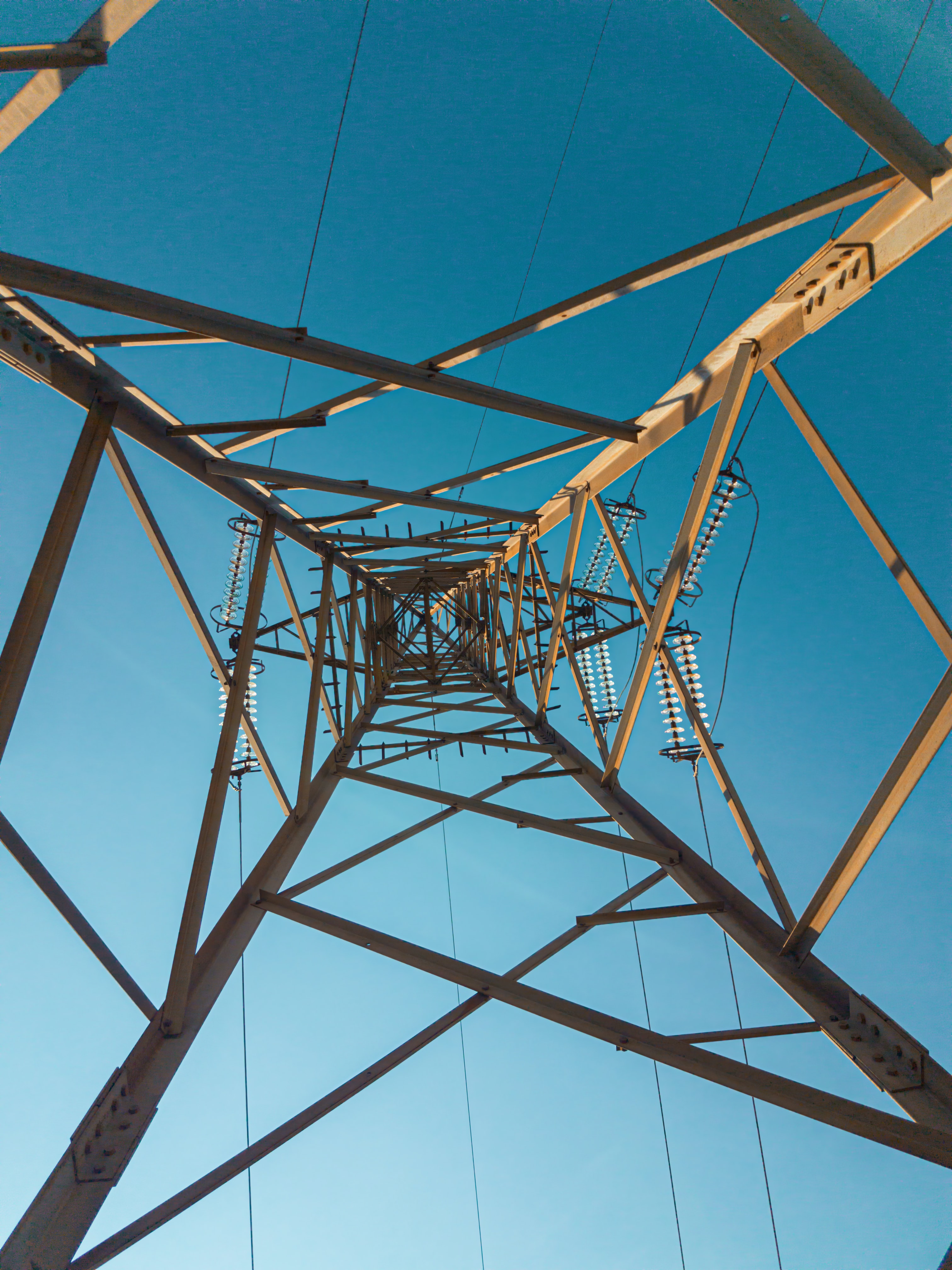
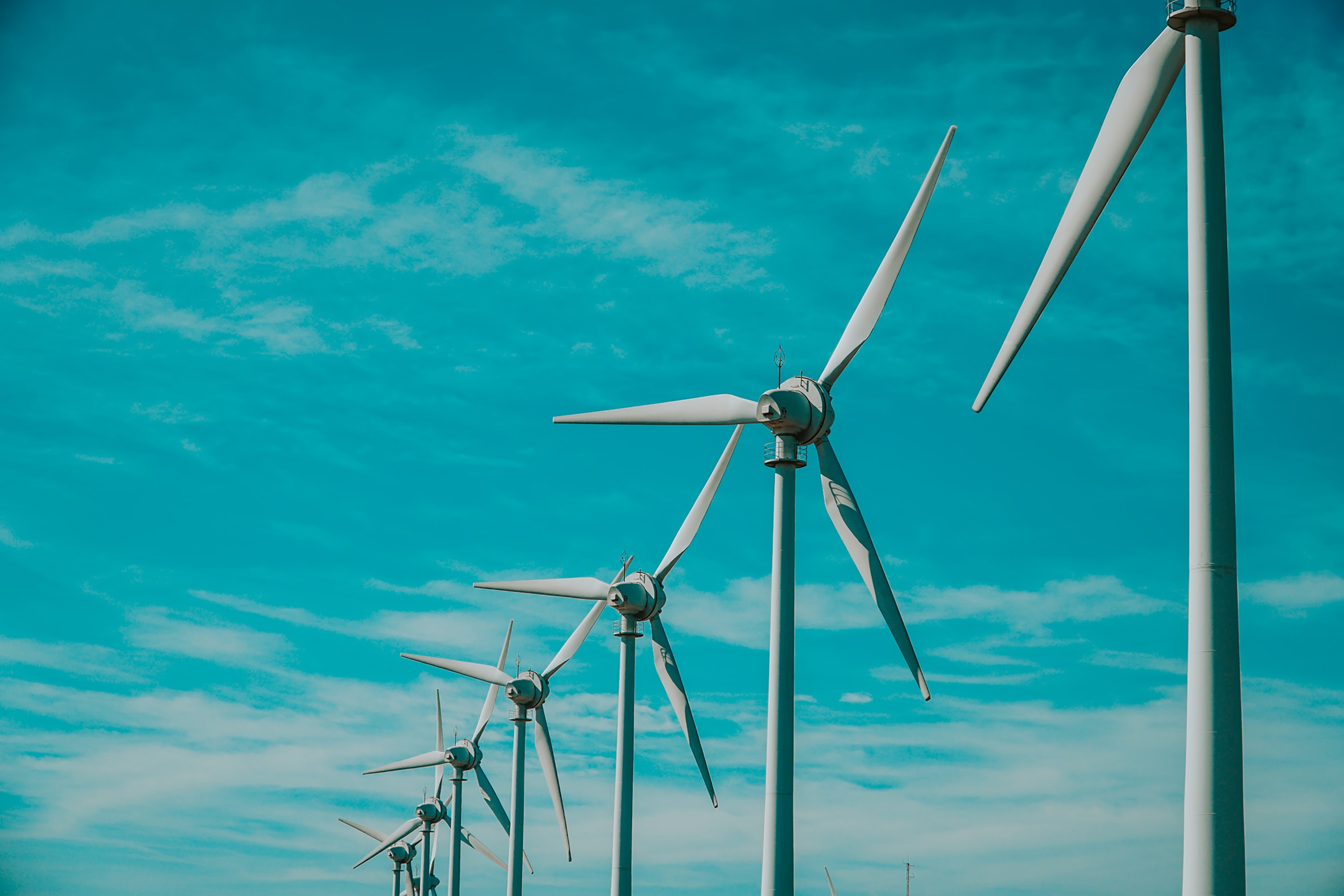
AI for research and innovation
By analysing complex datasets and uncovering previously unknown patterns, machine learning has the potential to accelerate scientific discovery across the sciences – from healthcare to climate science, fundamental physics to conservation, and more. Realising this potential requires action to equip researchers from across disciplines with the skills they need to use machine learning in their work, and to build a community of practice at the interface of data science and other disciplines. Activities in this theme advance research, training, and engagement at the interface of AI and the sciences.
AI policy and data governance
Policy plays a crucial role in influencing where, how and for whose benefit machine learning systems are developed and deployed. Safe and reliable deployment of machine learning systems requires policy frameworks that embed trustworthy data governance; that promote the use of machine learning in areas where it has potential to improve public wellbeing; and that account for the wider implications of technological change on individuals and communities. Research in this theme considers what policy levers can shape the development of AI technologies.
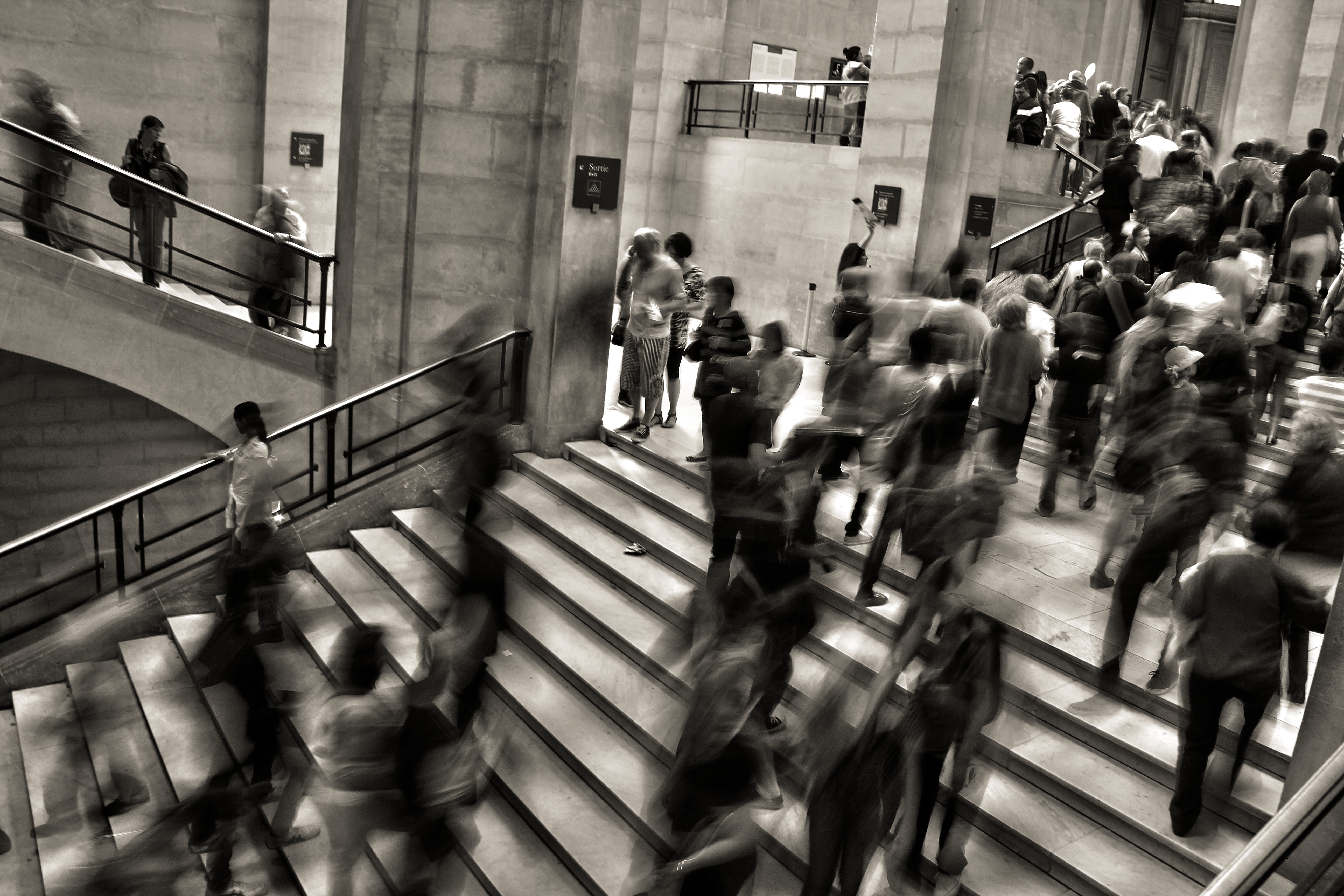
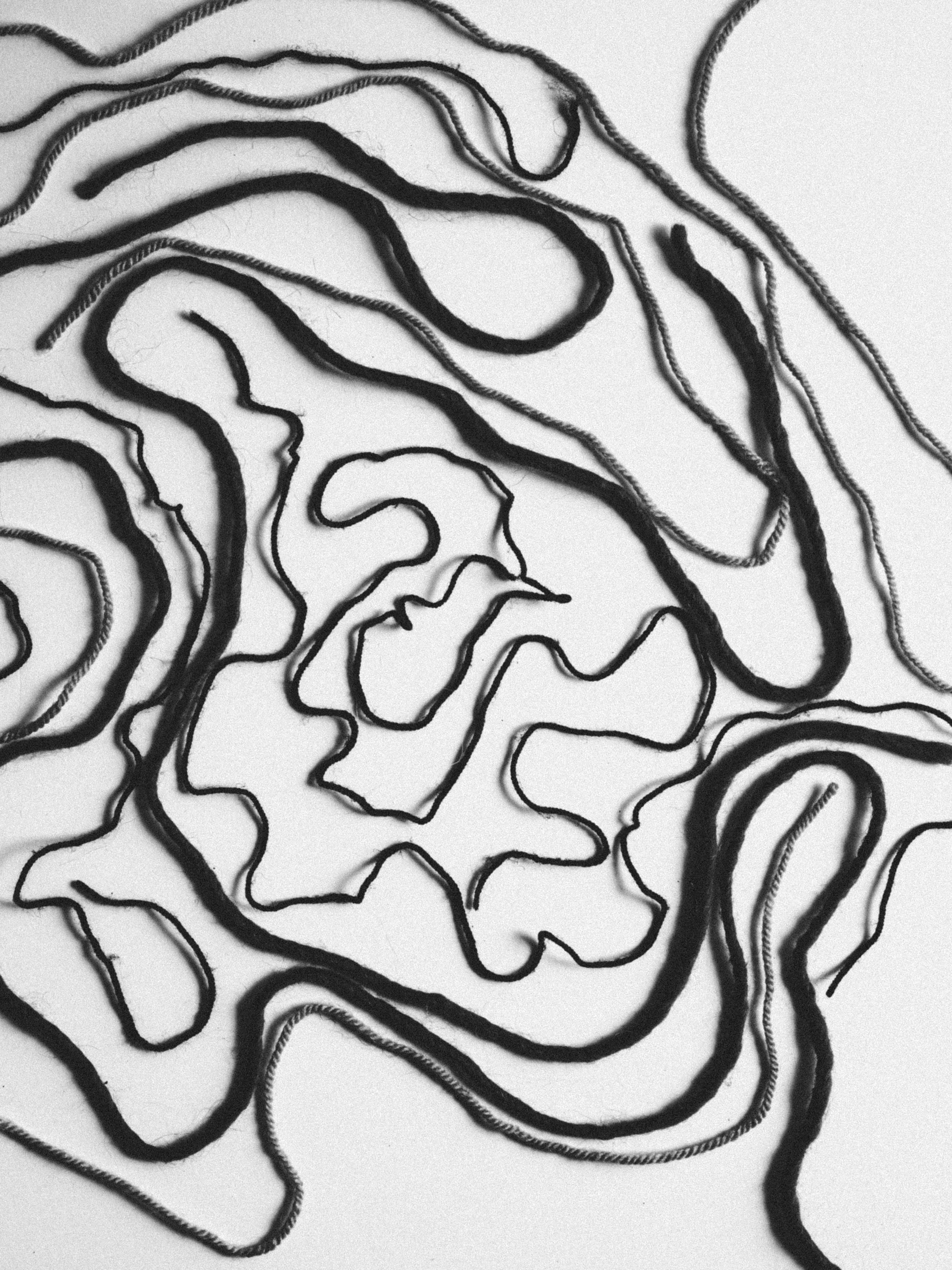
Machine learning theory and methods
Probability provides a language to describe our knowledge (or ignorance) of the world. To build statistical models, we need to structure our knowledge about a specific system such that we can reduce our ignorance using observations. These techniques are essential components for building interpretable computational structures that can be used for decision making. Research in this theme considers the development of probabilistic models and methods.