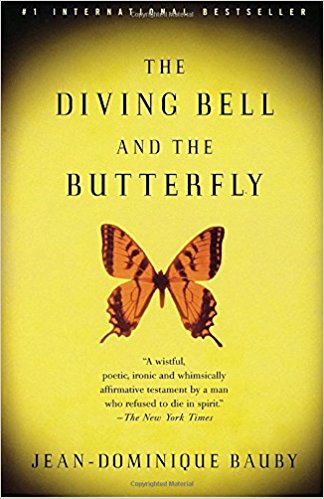
Topic : R255: Narratives in Artificial Intelligence and Machine Learning
Abstract:
In this talk we will explore a fundamental limitation of human intelligence which, we argue, makes cultural communication vital in sharing ideas. This will motivate the importance of narrative in setting cultural agenda.
Overview
In this topic we will explore the relationship between narrative and the development of machine learning and AI solutions. The course will be taught in collaboration with Nichole Anderson Ravindran and Alessandro Trevisan.
You can find text of Alessandro’s introduction to narratives here.
How do machine-learning researchers understand the narratives, philosophies, and theories surrounding the present-day understanding of AGI?
Can present-day and near-future concerns, issues, and themes be identified and extrapolated from future-oriented AI narratives?
We will look at look at different papers related to narratives, philosophies and theories related to artificial intelligence and determine if there are other meaningful ways of identifying problems, themes, and datasets curated based on them.
The first paper set is:
- Thousands of AI Authors on the Future of AI by Katja Grace, Harlan Stewart, Julia Fabienne Sandkühler, Stephen Thomas, Ben Weinstein-Raun, and Jan Brauner.
Here’s a list of other papers that could be chosen for other sessions, or if there’s something you’d like to suggest, let us know!
Asimov’s Laws of Robotics - Implications for Information Technology (Chapter 15 of Machine Ethics 2011; Cambridge Core) - Roger Clark
AI Armageddon and the Three Laws of Robotics (Ethics and Information Technology, Springer 2007) - Lee McCauley
The Three Laws of Robotics in the Age of Big Data (2016 Sidley Austin Distinguished Lecture on Big Data Law and Policy; Ohio State Law Journal) - Jack M. Balkin
The Unacceptability of Asimov’s Three Laws of Robotics as a Basis for Machine Ethics (Chapter 16 of Machine Ethics 2011; Cambridge Core) Susan Leigh Anderson
Monsters, Metaphors, and Machine Learning - (Proceedings of the 2020 CHI Conference on Human Factors in Computing Systems; April 23, 2020) - Graham Dove and Anne-Laure Fayard
Laplace’s Demon and the Adventures of His Apprentices (Cambridge Core, Philosophy of Science Journal, Jan. 1, 2022) - Roman Frigg, Seamus Bradley, Hailiang Du and Leonard A Smith
Studying Computer Scientists (Chapter 1 of The Constitution of Algorithms; MIT Press, 2020) - Florian Jaton
AGI Ruin: A List of Lethalities (2022 MIRI Alignment Discussion; Blogpost, LessWrong, June 5, 2022) - Eliezer Yudkowsky
Meditations on Moloch Meditations on Moloch (Reflective Essay written by Scott Alexander on Slate Star Codex, July 30th, 2014.)
The Artificial Intelligence of the Ethics of Artificial Intelligence - An Introductory Overview for Law and Regulations (Chapter 1 of The Oxford Handbook of Ethics of AI, 2020) - Edited by Markus D. Dubber, Frank Pasquale, and Sunit Das
Could a Large Language Model be Conscious? (arXiv; April 29, 2023)David J. Chalmers
Do Machine-Learning Machines Learn (Conference Paper in Philosophy and Theory of Artificial Intelligence 2017; SAPERE Vol. 44; August 29, 2018) - Selmer Bringsjord, Naveen Sundar Govindarajulu, Shreya Banerjee, and John Hummel
AI Risk Skepticism (Conference Paper in Philosophy and Theory of Artificial Intelligence 2021; SAPERE Vol. 63; November 15, 2022) - Roman V. Yampolskiy
Toward Out-of-Distribution Generalization Through Inductive Biases (Conference Paper in Philosophy and Theory of Artificial Intelligence 2021; SAPERE Vol. 63; November 15, 2022) - Caterina Moruzzi
The Vulnerability World Hypothesis (Global Policy Vol. 10; November 2019) - Nick Bostrom
AI is Like…A Literature Review of AI Metaphors and Why They Matter for Policy (AI Foundations Report#2, Legal Priorities Project, Oct. 2023) - Matthijs M. Maas
Introduction - How Chinese Philosophers Think about Artificial Intelligence (Introduction of Intelligence and Wisdom - Artificial Intelligence Meets Chinese Philosophers, Edited by Bing Song, Sept. 2021) - Bing Song
The Artificial Intelligence Challenge and the End of Humanity (3rd Chapter Introduction of Intelligence and Wisdom - Artificial Intelligence Meets Chinese Philosophers, Edited by Bing Song, Sept. 2021) - Chenyang Li
The Uncertain Gamble of Infinite Technological Progress (10th Chapter of Introduction of Intelligence and Wisdom - Artificial Intelligence Meets Chinese Philosophers, Edited by Bing Song, Sept. 2021) - Tingyang Zhao
Artificial Intelligence and African Conceptions of Personhood (Ethics and Information Technology, Vol. 23(2), pgs 127-136, June 2021; originally published June 2020) - C.S. Wareham
Decolonial AI - Decolonial Theory as Sociotechnical Foresight in Artificial Intelligence (Philosophy & Technology, Vol. 33, pgs 659-684, July 2020) - Shakir Mohamed, Marie-Therese Png, and William Isaac
The presentation for each paper will be in the form of an 800 word summary that captures the main message of the work and sets it against the context of the wider discussion we will set up in this first session.
The Diving Bell and the Butterfly
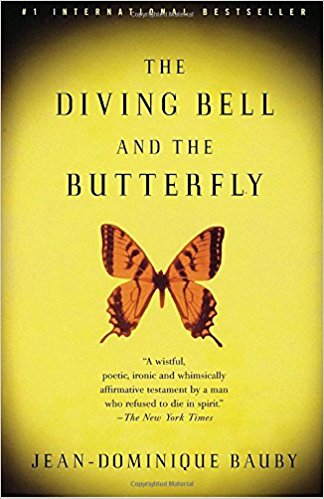
Figure: The Diving Bell and the Buttefly is the autobiography of Jean Dominique Bauby.
The Diving Bell and the Butterfly is the autobiography of Jean Dominique Bauby. Jean Dominique, the editor of French Elle magazine, suffered a major stroke at the age of 43 in 1995. The stroke paralyzed him and rendered him speechless. He was only able to blink his left eyelid, he became a sufferer of locked in syndrome.
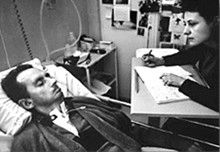
Figure: Jean Dominique Bauby was the Editor in Chief of the French Elle Magazine, he suffered a stroke that destroyed his brainstem, leaving him only capable of moving one eye. Jean Dominique became a victim of locked in syndrome.
Incredibly, Jean Dominique wrote his book after he became locked in. It took him 10 months of four hours a day to write the book. Each word took two minutes to write.
The idea behind embodiment factors is that we are all in that situation. While not as extreme as for Bauby, we all have somewhat of a locked in intelligence.
Embodiment Factors
![]() |
|||
bits/min | billions | 2000 | 6 |
billion calculations/s |
~100 | a billion | a billion |
embodiment | 20 minutes | 5 billion years | 15 trillion years |
Figure: Embodiment factors are the ratio between our ability to compute and our ability to communicate. Jean Dominique Bauby suffered from locked-in syndrome. The embodiment factors show that relative to the machine we are also locked in. In the table we represent embodiment as the length of time it would take to communicate one second’s worth of computation. For computers it is a matter of minutes, but for a human, whether locked in or not, it is a matter of many millions of years.
Let me explain what I mean. Claude Shannon introduced a mathematical concept of information for the purposes of understanding telephone exchanges.
Information has many meanings, but mathematically, Shannon defined a bit of information to be the amount of information you get from tossing a coin.
If I toss a coin, and look at it, I know the answer. You don’t. But if I now tell you the answer I communicate to you 1 bit of information. Shannon defined this as the fundamental unit of information.
If I toss the coin twice, and tell you the result of both tosses, I give you two bits of information. Information is additive.
Shannon also estimated the average information associated with the English language. He estimated that the average information in any word is 12 bits, equivalent to twelve coin tosses.
So every two minutes Bauby was able to communicate 12 bits, or six bits per minute.
This is the information transfer rate he was limited to, the rate at which he could communicate.
Compare this to me, talking now. The average speaker for TEDX speaks around 160 words per minute. That’s 320 times faster than Bauby or around a 2000 bits per minute. 2000 coin tosses per minute.
But, just think how much thought Bauby was putting into every sentence. Imagine how carefully chosen each of his words was. Because he was communication constrained he could put more thought into each of his words. Into thinking about his audience.
So, his intelligence became locked in. He thinks as fast as any of us, but can communicate slower. Like the tree falling in the woods with no one there to hear it, his intelligence is embedded inside him.
Two thousand coin tosses per minute sounds pretty impressive, but this talk is not just about us, it’s about our computers, and the type of intelligence we are creating within them.
So how does two thousand compare to our digital companions? When computers talk to each other, they do so with billions of coin tosses per minute.
Let’s imagine for a moment, that instead of talking about communication of information, we are actually talking about money. Bauby would have 6 dollars. I would have 2000 dollars, and my computer has billions of dollars.
The internet has interconnected computers and equipped them with extremely high transfer rates.
However, by our very best estimates, computers actually think slower than us.
How can that be? You might ask, computers calculate much faster than me. That’s true, but underlying your conscious thoughts there are a lot of calculations going on.
Each thought involves many thousands, millions or billions of calculations. How many exactly, we don’t know yet, because we don’t know how the brain turns calculations into thoughts.
Our best estimates suggest that to simulate your brain a computer would have to be as large as the UK Met Office machine here in Exeter. That’s a 250 million pound machine, the fastest in the UK. It can do 16 billion billon calculations per second.
It simulates the weather across the word every day, that’s how much power we think we need to simulate our brains.
So, in terms of our computational power we are extraordinary, but in terms of our ability to explain ourselves, just like Bauby, we are locked in.
For a typical computer, to communicate everything it computes in one second, it would only take it a couple of minutes. For us to do the same would take 15 billion years.
If intelligence is fundamentally about processing and sharing of information. This gives us a fundamental constraint on human intelligence that dictates its nature.
I call this ratio between the time it takes to compute something, and the time it takes to say it, the embodiment factor (Lawrence, 2017). Because it reflects how embodied our cognition is.
If it takes you two minutes to say the thing you have thought in a second, then you are a computer. If it takes you 15 billion years, then you are a human.
Human Communication
For human conversation to work, we require an internal model of who we are speaking to. We model each other, and combine our sense of who they are, who they think we are, and what has been said. This is our approach to dealing with the limited bandwidth connection we have. Empathy and understanding of intent. Mental dispositional concepts are used to augment our limited communication bandwidth.
Fritz Heider referred to the important point of a conversation as being that they are happenings that are “psychologically represented in each of the participants” (his emphasis) (Heider, 1958).
Bandwidth Constrained Conversations
Figure: Conversation relies on internal models of other individuals.
Figure: Misunderstanding of context and who we are talking to leads to arguments.
Embodiment factors imply that, in our communication between humans, what is not said is, perhaps, more important than what is said. To communicate with each other we need to have a model of who each of us are.
To aid this, in society, we are required to perform roles. Whether as a parent, a teacher, an employee or a boss. Each of these roles requires that we conform to certain standards of behaviour to facilitate communication between ourselves.
Control of self is vitally important to these communications.
The high availability of data available to humans undermines human-to-human communication channels by providing new routes to undermining our control of self.
A Six Word Novel
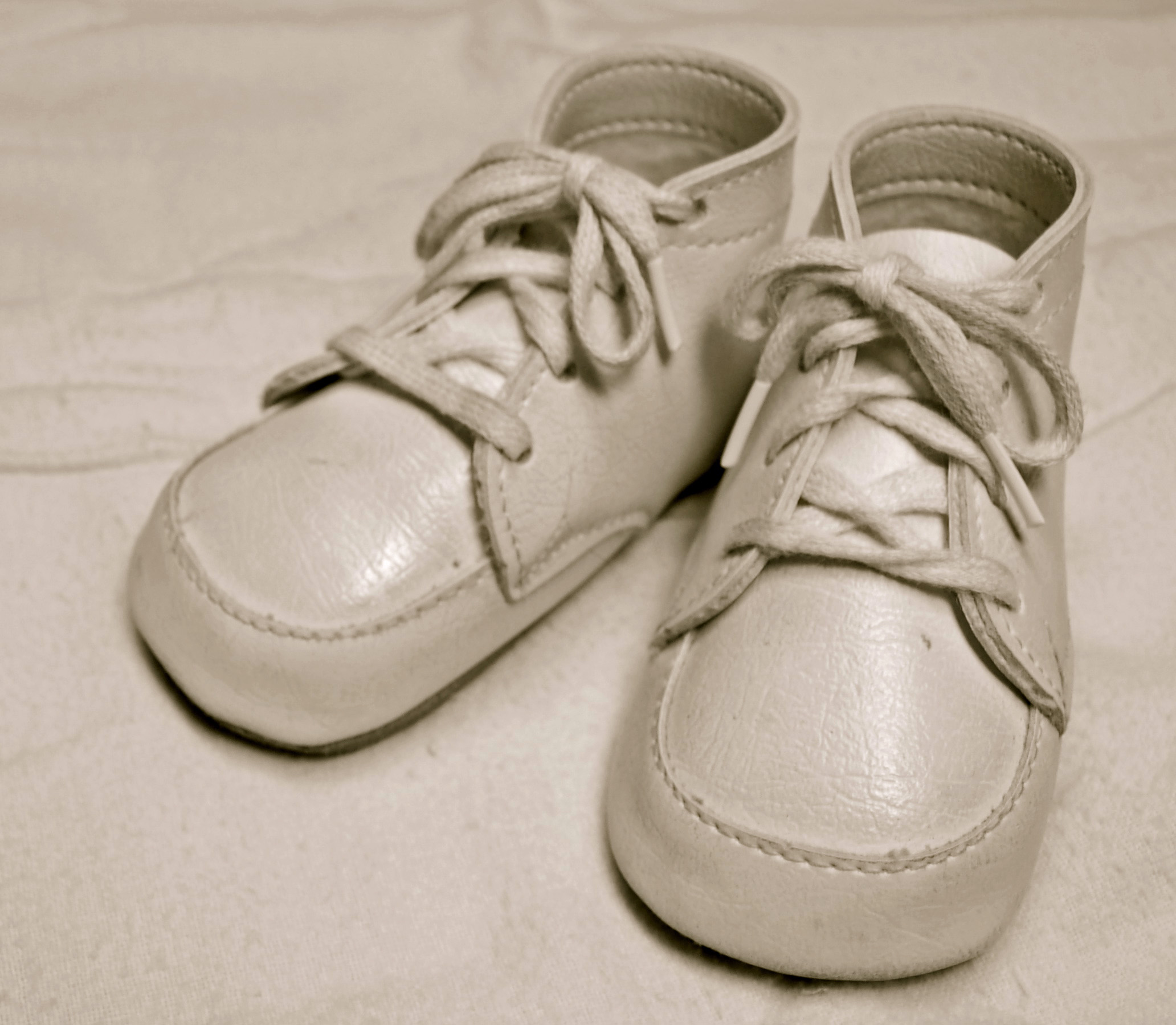
Figure: Consider the six-word novel, apocryphally credited to Ernest Hemingway, “For sale: baby shoes, never worn”. To understand what that means to a human, you need a great deal of additional context. Context that is not directly accessible to a machine that has not got both the evolved and contextual understanding of our own condition to realize both the implication of the advert and what that implication means emotionally to the previous owner.
But this is a very different kind of intelligence than ours. A computer cannot understand the depth of the Ernest Hemingway’s apocryphal six-word novel: “For Sale, Baby Shoes, Never worn”, because it isn’t equipped with that ability to model the complexity of humanity that underlies that statement.
Evolved Relationship with Information
The high bandwidth of computers has resulted in a close relationship between the computer and data. Large amounts of information can flow between the two. The degree to which the computer is mediating our relationship with data means that we should consider it an intermediary.
Originally our low bandwidth relationship with data was affected by two characteristics. Firstly, our tendency to over-interpret driven by our need to extract as much knowledge from our low bandwidth information channel as possible. Secondly, by our improved understanding of the domain of mathematical statistics and how our cognitive biases can mislead us.
With this new set up there is a potential for assimilating far more information via the computer, but the computer can present this to us in various ways. If its motives are not aligned with ours then it can misrepresent the information. This needn’t be nefarious it can be simply because of the computer pursuing a different objective from us. For example, if the computer is aiming to maximize our interaction time that may be a different objective from ours which may be to summarize information in a representative manner in the shortest possible length of time.
For example, for me, it was a common experience to pick up my telephone with the intention of checking when my next appointment was, but to soon find myself distracted by another application on the phone and end up reading something on the internet. By the time I’d finished reading, I would often have forgotten the reason I picked up my phone in the first place.
There are great benefits to be had from the huge amount of information we can unlock from this evolved relationship between us and data. In biology, large scale data sharing has been driven by a revolution in genomic, transcriptomic and epigenomic measurement. The improved inferences that can be drawn through summarizing data by computer have fundamentally changed the nature of biological science, now this phenomenon is also influencing us in our daily lives as data measured by happenstance is increasingly used to characterize us.
Better mediation of this flow requires a better understanding of human-computer interaction. This in turn involves understanding our own intelligence better, what its cognitive biases are and how these might mislead us.
For further thoughts see Guardian article on marketing in the internet era from 2015.
You can also check my blog post on System Zero. This was also written in 2015.
New Flow of Information
Classically the field of statistics focused on mediating the relationship between the machine and the human. Our limited bandwidth of communication means we tend to over-interpret the limited information that we are given, in the extreme we assign motives and desires to inanimate objects (a process known as anthropomorphizing). Much of mathematical statistics was developed to help temper this tendency and understand when we are valid in drawing conclusions from data.
Figure: The trinity of human, data, and computer, and highlights the modern phenomenon. The communication channel between computer and data now has an extremely high bandwidth. The channel between human and computer and the channel between data and human is narrow. New direction of information flow, information is reaching us mediated by the computer. The focus on classical statistics reflected the importance of the direct communication between human and data. The modern challenges of data science emerge when that relationship is being mediated by the machine.
Data science brings new challenges. In particular, there is a very large bandwidth connection between the machine and data. This means that our relationship with data is now commonly being mediated by the machine. Whether this is in the acquisition of new data, which now happens by happenstance rather than with purpose, or the interpretation of that data where we are increasingly relying on machines to summarize what the data contains. This is leading to the emerging field of data science, which must not only deal with the same challenges that mathematical statistics faced in tempering our tendency to over interpret data but must also deal with the possibility that the machine has either inadvertently or maliciously misrepresented the underlying data.
Computer Conversations
Figure: Conversation relies on internal models of other individuals.
Figure: Misunderstanding of context and who we are talking to leads to arguments.
Similarly, we find it difficult to comprehend how computers are making decisions. Because they do so with more data than we can possibly imagine.
In many respects, this is not a problem, it’s a good thing. Computers and us are good at different things. But when we interact with a computer, when it acts in a different way to us, we need to remember why.
Just as the first step to getting along with other humans is understanding other humans, so it needs to be with getting along with our computers.
Embodiment factors explain why, at the same time, computers are so impressive in simulating our weather, but so poor at predicting our moods. Our complexity is greater than that of our weather, and each of us is tuned to read and respond to one another.
Their intelligence is different. It is based on very large quantities of data that we cannot absorb. Our computers don’t have a complex internal model of who we are. They don’t understand the human condition. They are not tuned to respond to us as we are to each other.
Embodiment factors encapsulate a profound thing about the nature of humans. Our locked in intelligence means that we are striving to communicate, so we put a lot of thought into what we’re communicating with. And if we’re communicating with something complex, we naturally anthropomorphize them.
We give our dogs, our cats, and our cars human motivations. We do the same with our computers. We anthropomorphize them. We assume that they have the same objectives as us and the same constraints. They don’t.
This means, that when we worry about artificial intelligence, we worry about the wrong things. We fear computers that behave like more powerful versions of ourselves that will struggle to outcompete us.
In reality, the challenge is that our computers cannot be human enough. They cannot understand us with the depth we understand one another. They drop below our cognitive radar and operate outside our mental models.
The real danger is that computers don’t anthropomorphize. They’ll make decisions in isolation from us without our supervision because they can’t communicate truly and deeply with us.
Thanks!
For more information on these subjects and more you might want to check the following resources.
- twitter: @lawrennd
- podcast: The Talking Machines
- newspaper: Guardian Profile Page
- blog: http://inverseprobability.com